ObjectStyler Savva Kolbachev wins 3rd prize in Computer Vision contest by Chimp&See
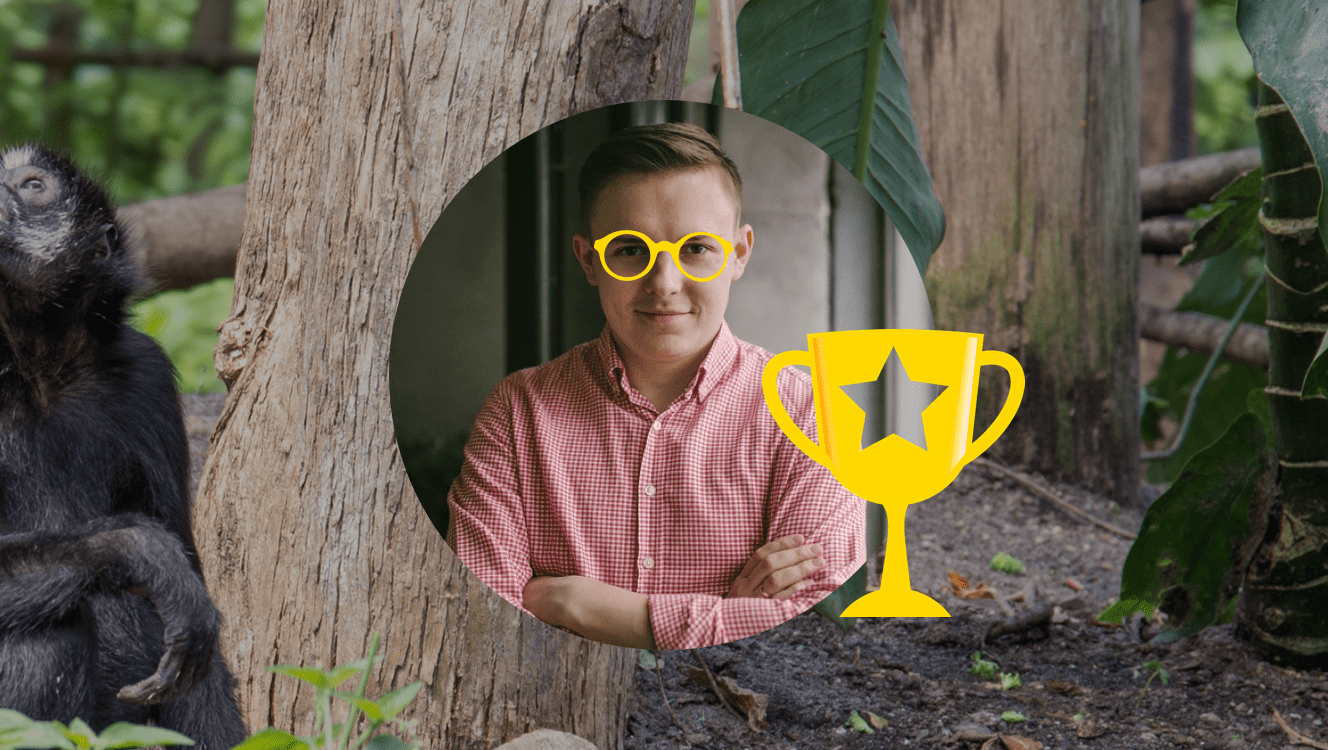
Evolutionary anthropology is a science that studies human origins. Among other things, it takes interest in the behavior of primates, such as chimpanzees, because it can help us understand how the earliest hominids evolved.
The Chimp&See project is hours upon hours of wildlife footage from so-called “camera traps” situated across West and Central Africa. But there’s one problem: there’s 1TB+ of unclassified recordings and no way of telling which ones have chimpanzees in them.
That’s why the Max Planck Institute of Evolutionary Anthropology called on the global community of data scientists for help. At the turn of 2018, the institute hosted an international contest (organized by DrivenData Labs) with the purpose of finding a programmatic solution to identifying chimps in the vast corpus of videos.
Training machines to spot chimps
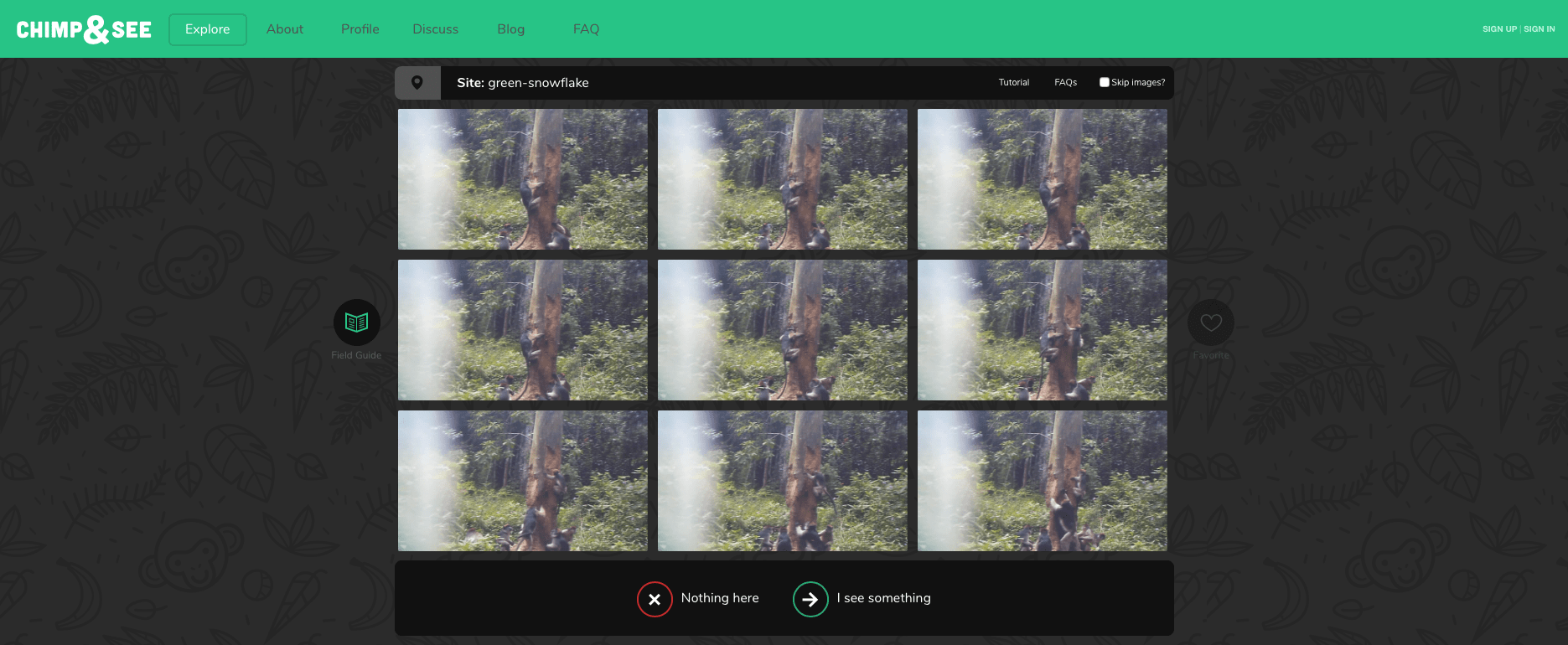
The rules of the contest were as follows: the contestant/team whose solution has the smallest aggregated logarithmic loss across all submitted models wins one of the three prizes. In plain words, the model should strive to do its best to spot videos with chimps.
The model is, basically, a machine-trained algorithm that can automatically tell if a given video contains any animals, and what kind of animal(s) they are. All in all, the contestants had to deal with 23 animal categories, plus 1 category corresponding to no animal.
The results
The competition turned out to be a heated struggle of brilliant minds that also included some intrigue and drama.
While the first two prizes got secured by other contestants, the 3rd prize went to ObjectStyler Savva Kolbachev and his teammate Alexander Veysov, who were able to identify chimps with 90%+ accuracy – congrats!
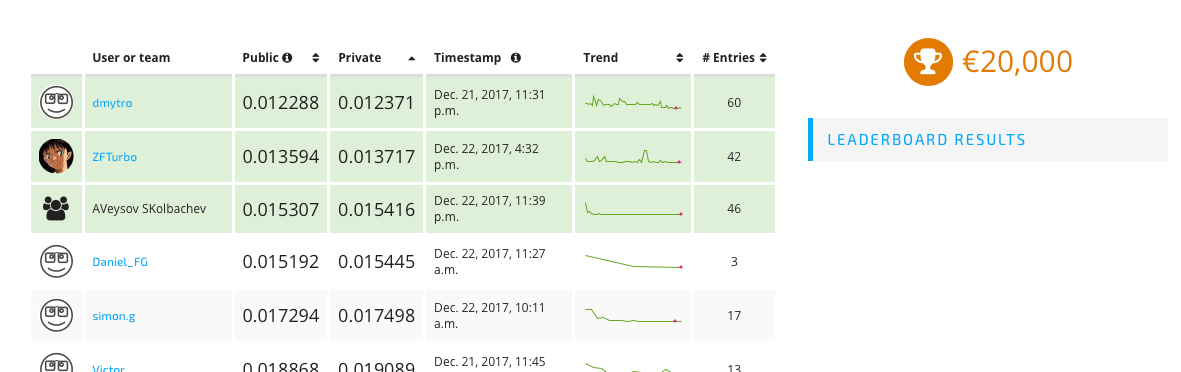
Psst… the code for their solution is available on GitHub.