Discussion: Do Humans Expect Too Much of AI?
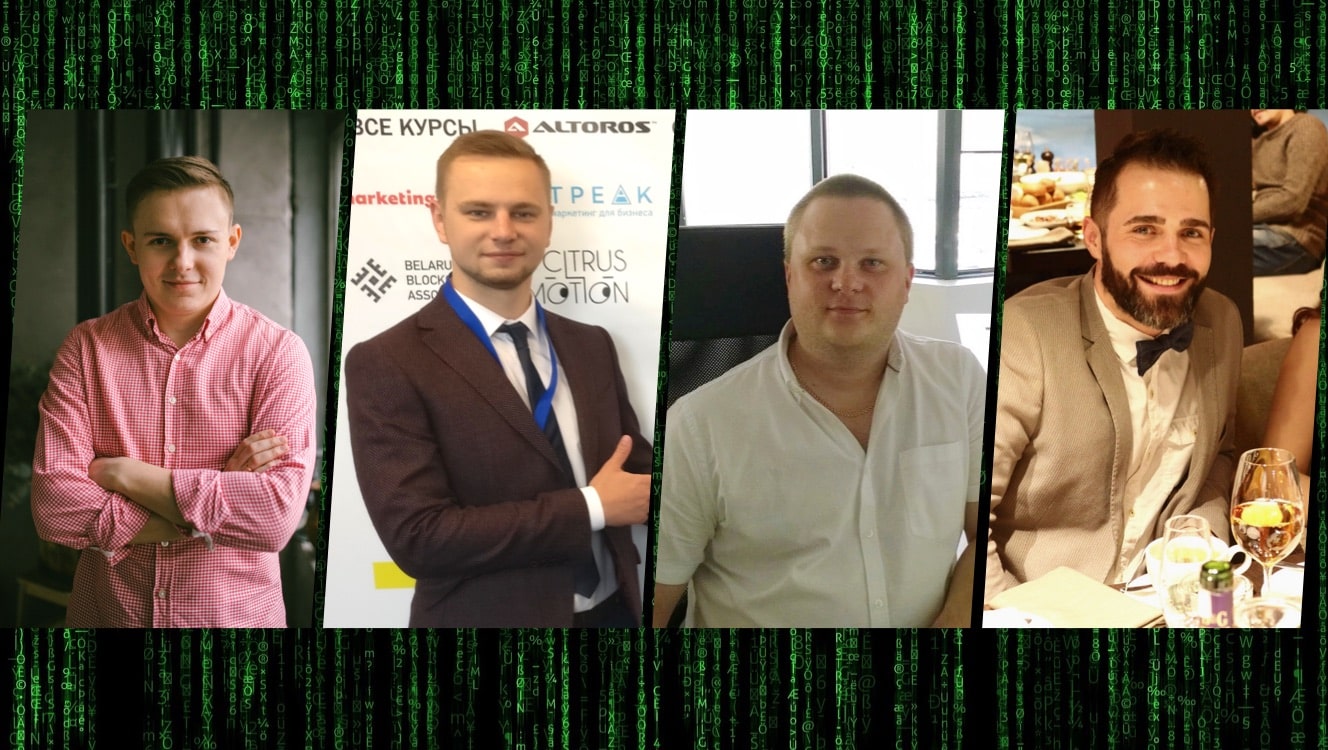
We have a Data Science Lab here at ObjectStyle. It serves to advance our AI knowledge and practical skills of implementing machine learning, among other things. One day, we decided to sit down and discuss the hype around AI, a possible “AI winter,” and likely economic ramifications of job automation. (“AI winter” is a time-period, during which AI is not developing fast due to certain reasons such as a lack of computing resources, or others.)
These three questions were brought up:
Q2: Is there anything holding back AI development now? Are there any unsolved technical challenges?
Q3: What political/economic obstacles to AI adoption do you see? Should governments be concerned about unemployment due to automation?
Here is who took part in this discussion:
Savva Kolbachev, Senior Data Scientist
Engeny Vintik, Senior Software Developer, Team Lead, ML enthusiast
Eduard Seredin, Senior Data Scientist
Anton Trukhanyonok, Enterprise Search Solution Engineer
Q1: Do you believe there’s too much hype around AI? Have you seen any exaggerated claims being made about the technology?
Savva Kolbachev: Some journalists describe things unrealistically, maybe because they don’t understand the technical part. They write: “Wow, a bot can now chat like a human,” but you know it’s nothing like that in reality. Or take this piece: Facebook is using ‘Lord of the Rings’ to teach its programs how to think. While the headline sounds impressive, underneath it all is a rather basic algorithm that serves to understand relationships between entities in a system. Not the kind of “thinking AI” you’d expect.
Marketers use catchy vocabulary to lure both users and investors, but when the solution fails to deliver, this may cause problems. AI is a broad concept that can stand for anything. Inside the bulk of AI technologies one finds machine learning, and inside ML one finds deep learning. But does a particular AI-powered solution use ML or DL? You never know.
AI is a broad concept that can stand for anything.
Anton Trukhanyonok: [draws a graph on the whiteboard]
In theory, all “hot” technologies go through Gartner’s hype cycle. A piece of tech looks extremely promising at first, at which point marketers start writing about it… they put out tons of articles and videos. Everyone wants in: startups, investors, and everybody else. Once the hype reaches its peak, it starts to go down fairly quickly. The reason for this is under-delivery; or, in other words, overly high expectations that could not be met.
When it comes to AI, some authors say we’re at the beginning of a nosedive at the moment. If AI proves viable in the long run, things will get balanced out. Like any mature technology, AI will be used in real-world scenarios where its use is justified. By contrast, during the hype stage, people try to squeeze the “hot” technology everywhere. We’ve seen this with blockchain, chatbots, “big data,” and a number of other hyped technologies. To sum it up, unrealistically high expectations are inevitably followed by under-delivery, which results in excessive skepticism followed by slow adoption in real-world applications.
Unrealistically high expectations are inevitably followed by under-delivery, which results in excessive skepticism followed by slow adoption in real-world applications.
Q2: Is there anything holding back AI development now? Are there any unsolved technical challenges?
Eduard Seredin: I’d like to give an example from the banking industry. It’s an area that’s highly interested in implementing AI for its needs. Predictive analytics has been around for a while, and it helps with a number of tasks, such as assessing the quality of a loan applicant. You may say that AI could take the game to a whole new level. However, even basic ML models are still missing mathematical proof nowadays. We expect them to make predictions like a human, but we’ve got no formal proof of the accuracy of these predictions.
Even basic ML models are still missing mathematical proof nowadays.
If or when mathematicians are able to come up with the formulae to substantialize ML models, we may see another surge in the development and adoption of AI technologies. In one survey, they asked bankers if they actually used predictive analytics available to them. Only 2-3% said they did. And only some 0.001% said they were ready to blindly trust automated predictions. That said, we suppose that robot-made assessments are accurate, but we cannot prove it. We can only suppose that they are.
Savva Kolbachev: I wouldn’t say there is anything holding back AI development right now. On the contrary – AI is in the best shape it’s ever been. Some people say there will be another “AI winter”, but I don’t think so. I believe this is just populist talk.
AI is in the best shape it’s ever been. Some people say there will be another “AI winter”, but I don’t think so. I believe this is just populist talk.
I do agree with Eduard that the theoretical base is not ready yet. Most of the recent discoveries are empirical rather than theoretical. But the computing resources available to Data Scientists have improved radically since the last ‘AI winter’. This, as well as availability of open-source solutions, made it much more accessible to a broader audience. A solution that was considered state-of-the-art yesterday is now feasible for a student with a laptop. So, there is still plenty of room for growth in AI.
Evgeny Vintik: In my opinion, the main limitations come from the very factors that drive AI research and development. AI is a relatively immature domain, and its gains are mostly consumed by the business sphere. Many solutions nowadays are discovered by trial and error within the boundaries of a specific project. Based on them, it’s hard to single out a universal AI solution that would be applicable across niches.
Many solutions nowadays are discovered by trial and error within the boundaries of a specific project. Based on them, it’s hard to single out a universal AI solution that would be applicable across niches.
For example, if you are solving a problem with image recognition, you can’t just apply the solution to speech recognition as a next logical step. That said, it’s unclear what’s the optimal strategy is. Should we strive to create general-purpose AI technologies? Or maybe holistic AI will be based on totally different methods in the future? We’ll live and see.
Eduard Seredin: Another barrier that really stands out to me is that, just like opensource, AI is a hodgepodge of languages and frameworks. The AI ecosystem leaves much to be desired in terms of compatibility of available solutions. Reusing your code and your solutions is a worldwide best practice. Still, it’s difficult to do in the case of AI.
There are too many moving parts. For instance, a company releases a new version of its video card. It’s difficult to understand what has changed unless you’re intimately familiar with the architecture. Now a whole bunch of solutions written for this video card, say, three years ago, will stop working. Assembling a working AI system with the currently available software and hardware components can be a daunting task, even for an experience engineer.
Assembling a working AI system with the currently available software and hardware components can be a daunting task, even for an experience engineer.
Q3: What political/economic obstacles to AI adoption do you see? Should governments be concerned about unemployment due to automation?
Savva Kolbachev: We’ve been there many times before. Every time a new technology appears, there are those who oppose it and say it’s the end of the world. There’s also a fair amount of lobbying. For instance, self-driving cars are facing lots of obstacles from law- and policy-makers. Their competitors that are not able to catch up try to lobby and curb self-driving alternatives.
At the same time, an ever-increasing number of states all across the world are putting forth legislative and other initiatives because they want to leverage the benefits of AI.
Eduard Seredin: A problem arises when you make changes all of a sudden. This applies not only to AI, but to any other sphere. If one day you come to work and they tell you, “Okay, pack up, because you’re being replaced with a robot,” of course that’s going to be a shock. But if companies plan for this in advance and if they let people prepare for the changes … let’s say, by learning a new trade or something, that is a different story.
All in all, I think it could be a good thing for everyone in the long run. We’ll finally get to do things for which we never have time otherwise. Such as learn something interesting, sleep more, take better care of our health. There will be more time for self-realization.
Anton Trukhanyonok: People like Karl Marx have been raising this question since the 18th century. I’m not an expert on this, but as things get automated, hands get freed up. And governments ask: “How do we occupy these people?” There are concerns that newly unemployed population will engage in antisocial behavior. But how is being unemployed worse than doing some mundane, mind-numbing job? And who says that people must work?
If you look at it from a philosophical POV, there is this “guaranteed basic income” idea when people don’t work and yet receive everything they need from the government. It’s unclear how this is supposed to work in reality. Maybe someone should be responsible for redistributing income from fully-automated factories.
Also, we still live in the consumption society where you need a new smartphone every year, a new laptop every couple of years, and a new car every five years. As material goods get ubiquitous, we may be in for an existential crisis, because we’ll need to come up with some new values and dreams to pursue. I don’t know what people will do … maybe we’ll invent some higher-purpose philosophy or something like that.
Many thanks to our Data Science Lab for sharing their opinions! If you have something to say on these points, share your thoughts in the comments. Thank you!
Related Blogs
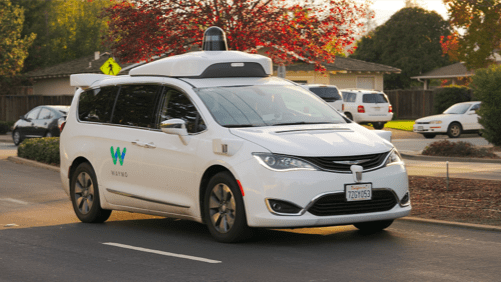
Why making driverless cars is hard - and why you have to may wait indefinitely for them
LEARN MORE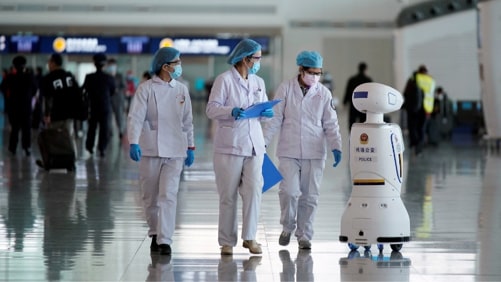