Why making driverless cars is hard – and why you may have to wait indefinitely for them
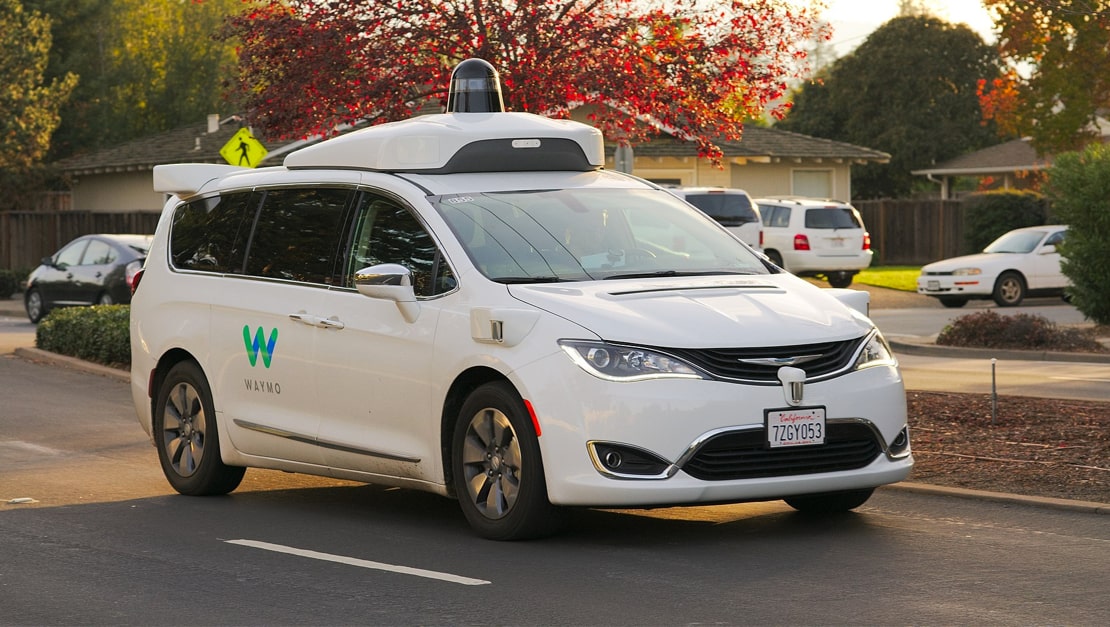
The promise of the driverless car is huge: it could free up a lot of time, spare us the need to get a driver’s license, improve road safety, empty our parking lots, or even replace car ownership.
But making self-driving cars is easier said than done. There still are quite a few technological and regulatory puzzles that need to be solved before AVs (autonomous vehicles) can be adopted on a mass scale. Let’s see what they are.
LiDAR sensors
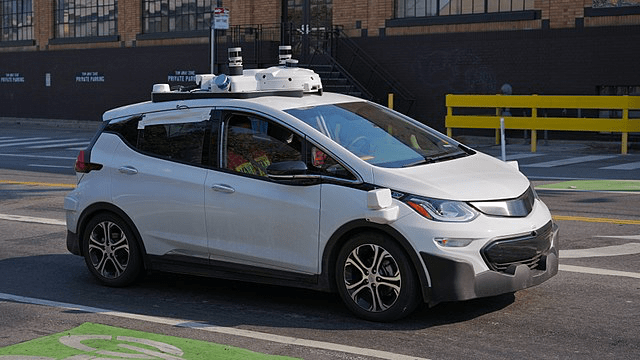
The LiDAR – or “light radar” – is an essential piece of tech that goes into most autonomous vehicles these days. It allows the car to “see” in 3D, ensuring it’s aware of the surroundings even in the dark.
But the problem with LiDARs is that they are extremely expensive: a top-of-the-range unit is priced around $75,000. This drives the cost of producing autonomous cars through the roof.
At the same time, not everyone views LiDARs as absolute necessity. Just recently, Tesla CEO Elon Musk voiced a radical opinion that “anyone who relied on LiDARs was doomed” (Tesla does not use LiDARs). Anthony Levandowski of Pronto.AI seconded that by saying that LiDARs were indeed “a crutch” and that the main problem with AVs was “not better lasers, but better prediction.”
That said, while big players like Google are now working their own cheaper LiDAR, others like Tesla are looking for ways to do without it.
Lithium-ion batteries
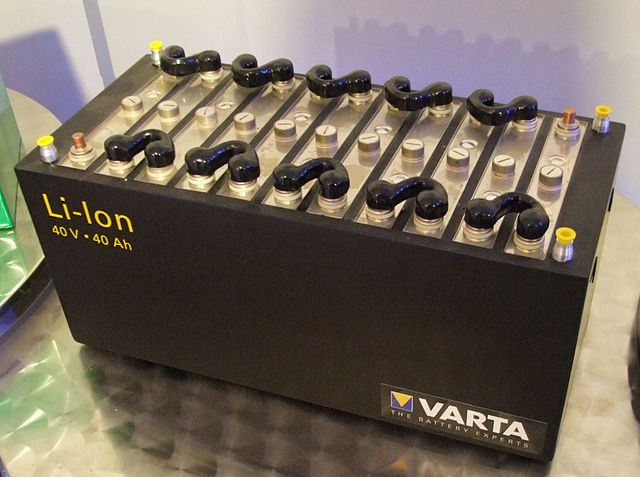
The world’s first prototype for a lithium-ion battery was created by the Japanese chemist Akira Yoshino back in 1985. Initially, the wonder batteries were used in smartphones and laptops. But now they also power electric cars.
Considering that a fair share of autonomous driving tech is being developed for electric cars (Tesla, Nissan LEAF, GM’s Chevrolet Bolt, etc.), they need energy-dense lithium-ion batteries to be able to roam the city for miles on end. These batteries are believed to strike a good balance between high performance and the low risk of overheating (although they can catch some pretty bad fire in some circumstances).
Now, the thing with the 20-pound car battery is that it’s much bigger than its iPhone version and needs significantly more cobalt – an essential ingredient in this type of battery. And that’s where it gets interesting. Two-thirds of the world’s cobalt reserves are in the Democratic Republic of Congo. Following the rise in EV production, cobalt prices went up significantly last year, causing carmakers to fall over one another in pursuit of better deals.
Panasonic, Tesla’s exclusive battery supplier, came under scrutiny when it was caught sourcing some of its cobalt from U.S.-sanctioned Cuba. After that, Panasonic said it was going to cut ties with its Canadian partner, who was the intermediary in the deal.
At the same time, Tesla and Panasonic have been working on a new type of battery that uses less cobalt – or, ideally, none at all. This looks like a necessary strategic move, because as the number of electric cars in the world grows, cobalt supplies may eventually get depleted.
Software: the missing piece
When it comes to self-driving cars, you’d expect the software to be nearly perfect by now. I mean, haven’t the code wizards at Alphabet/Google been working on their Waymo car for ten years already?
But the reality is, the artificial intelligence in present-day AVs is still far from perfect, and it still makes mistakes. Last month, AV tech pioneer Anthony Levandowski said in an interview:
In most cases, if you look at what went wrong during a disengagement [the moment when the AV needs human intervention – note by editor], the role of hardware failure is 0.0%. Most of the time, it’s a software failure, that is, software failing to predict what the vehicles are gonna be doing or what the pedestrians are gonna be doing.
Tesla’s Elon Musk echoed the opinion, saying that while AVs know what to do in many standard situations, existing simulations “do not capture the long tail of weird things that happen in the real world.”
And what are these simulations, exactly? They are virtual circumstances used by machine learning engineers to teach their AI models how to drive. It’s similar to how you’d teach a human: you show them what you would do in a concrete case – or a bunch of similar cases – and the person learns to imitate your behavior.
Besides the simulation training, real-life data is extremely valuable, too. Here’s where companies that were able to collect the most real-life statistics have an advantage.
Selected AV makers: their software and testing approaches
Brand | Car Make | Software | Testing | Miles driven |
---|---|---|---|---|
Alphabet’s Waymo | Chrysler Pacifica | Proprietary software | -The Carcraft simulation system. -On public roads in 25 cities in California and Arizona. |
Last year: -1.2 million miles in the real world All time: -5 billion miles in simulation -10 million miles in the real world |
General Motors (GM Cruise) | Chevy Bolt | The end-to-end Cruise platform | -The Matrix simulation with 30,000 virtual cars -On public roads in California |
Last year: -450,000 miles in the real world -n/a miles driven in simulation* |
*GM Cruise does not measure miles driven in their Matrix simulation, as it doesn’t consider the metric useful. | ||||
Tesla | Model S, Model X, Model 3, and others | -Proprietary software -Semi-autonomous Driver Assist is already in use |
-500,000 real-life cars are used in “shadow testing”* | An estimated 1.35 million miles driven on autopilot in the real world |
*Tesla’s “shadow testing” is different from both simulation and test vehicle miles, because it includes miles driven by actual drivers anywhere on the road in non-geofenced areas. | ||||
Baidu | Ford, Volvo, BAIC (Beijing Hyundai and Beijing Benz), and others | The Apollo platform | On public roads in Beijing and California | Last year: 87,000 miles in the real world in Beijing |
Volvo | Volvo XC90 | Zenuity software | About 100 cars on public roads in Sweden | N/A |
Uber | Refitted Volvo XC90 | Proprietary software by Uber’s Advanced Technologies Group (ex-Otto) | 250 autonomous vehicles tested on California’s public roads (previously – in Arizona) | Millions of real-world testing miles and 10,000s passenger trips claimed |
Nissan | Nissan Leaf, Infiniti Q50 | – Proprietary software -Semi-autonomous ProPILOT system already in use |
On public roads around Tokyo. Previously – in London and California. | N/A |
Volkwagen’s Audi | Audi A8, VW Golf | -Autonomous Intelligent Driving (AID) -The Aurora platform -Semi-autonomous Traffic Jam Pilot already in use |
Reportedly, 12 autonomous vehicles on public roads in Munich | N/A |
Ford | Ford Fusion | Argo AI | Up to 100 cars by the end of 2019; testing in Miami, Washington D.C., and Detroit |
N/A |
Aptiv | Renault Zoe, BMW, Peugeot, Audi, and others | The nuCore™ platform | Testing in Las Vegas via the Lyft app; may be coming to Beijing soon |
40,000 paid autonomous rides completed in Las Vegas |
Open source could be the answer
The success of Linux and OpenStreetMap demonstrates that the power of open-source can revolutionize a technology space. Now, many AV players are hoping to step up their research and development efforts through open-source.
Leading the way here is Baidu, China’s version of Google. In the spring of 2017, Baidu announced the Apollo initiative, which is sometimes dubbed “Android for autonomous vehicles.” The platform offers open-source software, an open simulation engine, and an autonomous driving algorithm that has “the world’s largest volume of deep-learning data sets.”
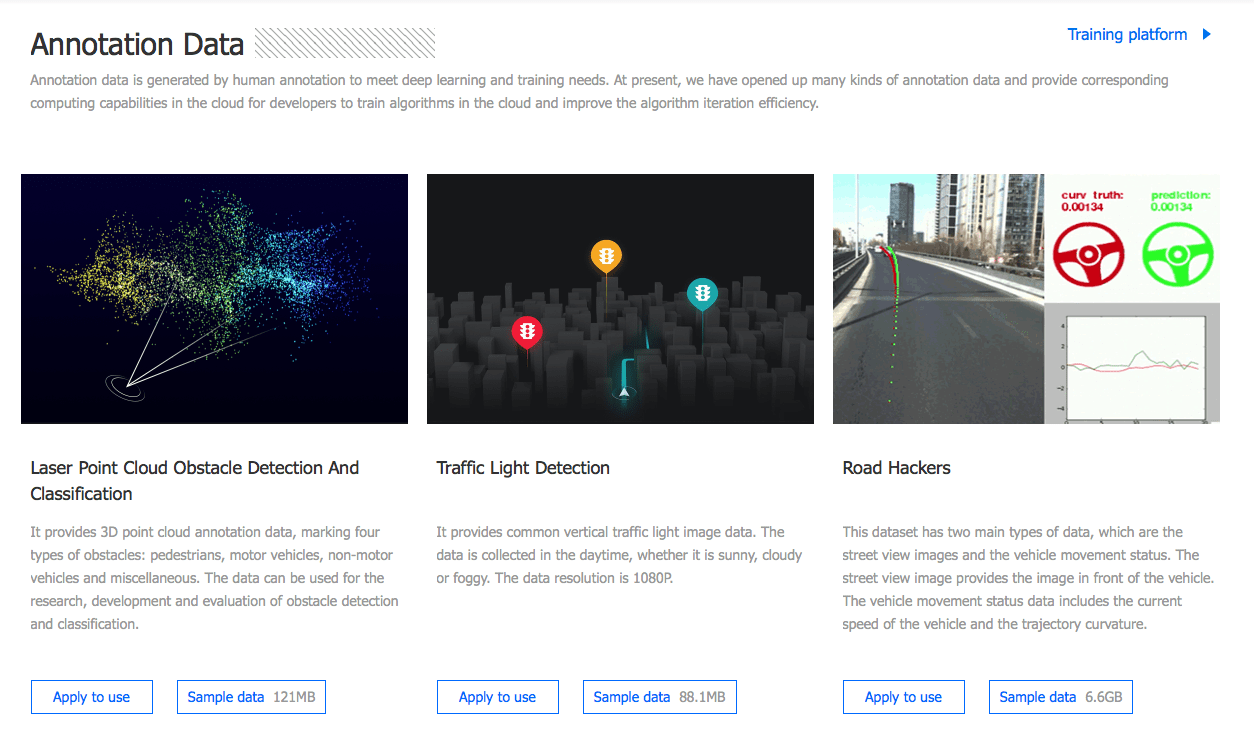
In just two years since its launch, the consortium has attracted some high-profile members, including Ford, Volkswagen, BMW, Hyundai, Honda, Volvo, and Daimler.
But Baidu isn’t the only company to have come forward with this type of initiative. Aptiv, which many consider a player to watch, recently unveiled nuScenes, which is an open-source dataset for autonomous driving.
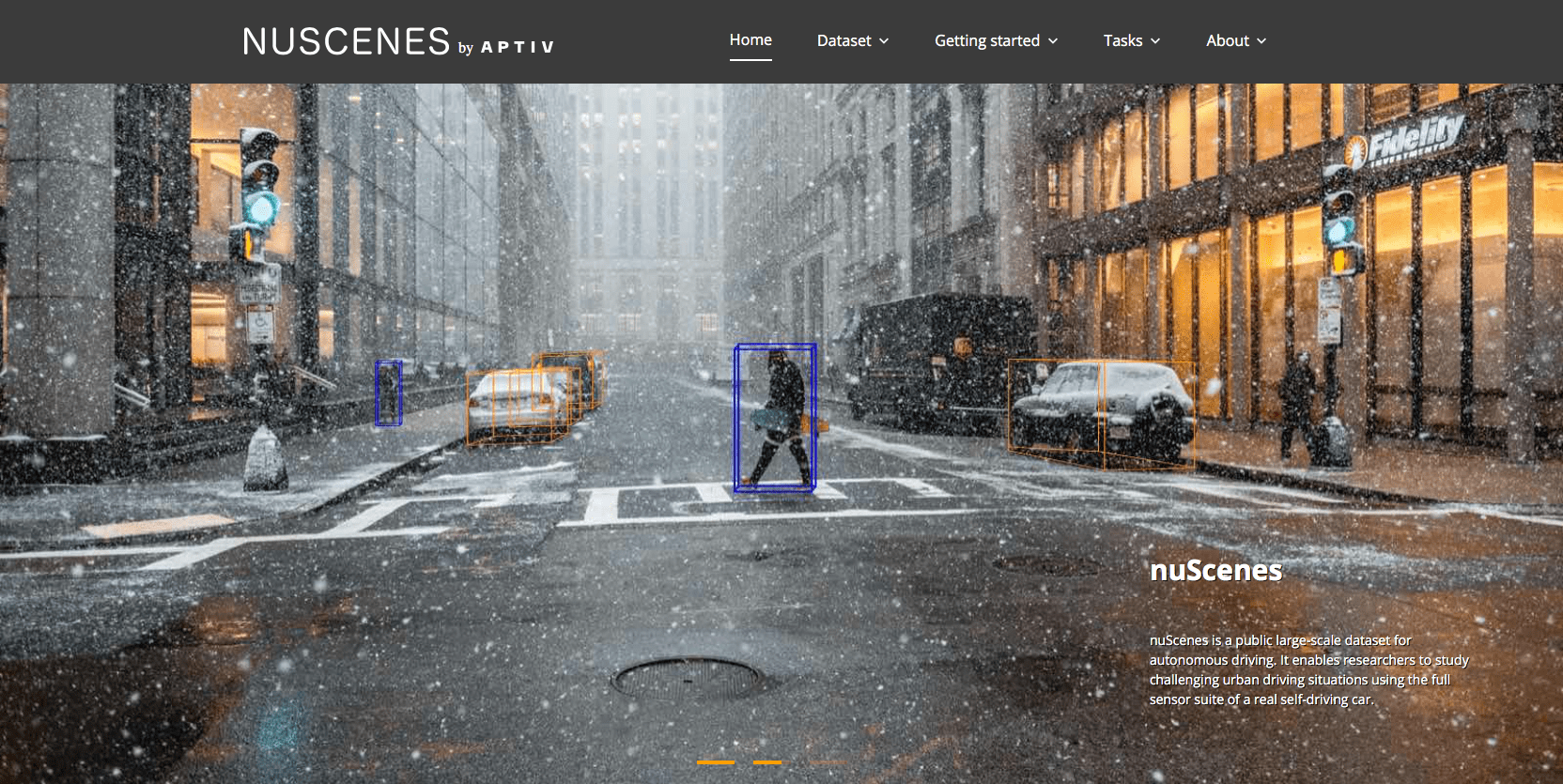
Although autonomous vehicle technology has made significant progress by now, legislative hurdles and public pushback still remain that may further delay AV arrival. Many in the industry believe initial implementation will probably be geo-fenced, taxi service-oriented, and possibly limited in other ways.
In conclusion
Making autonomous cars is a complex process, so it doesn’t seem feasible to analyze all existing difficulties in one post. There are just too many facets involved: car manufacturing, cartography, chips, sensors, radars, AI software, safety research, legislative acts, and even chemistry. Therefore, we tried to research just the issues that stick out.
Considering there are many challenges to overcome, the industry may take a while to deliver a safe, fully driverless car. And we’ll wait and see when that happens.
Lead image credit: Dllu, CC BY-SA 4.0, via Wikimedia Commons
Related Blogs
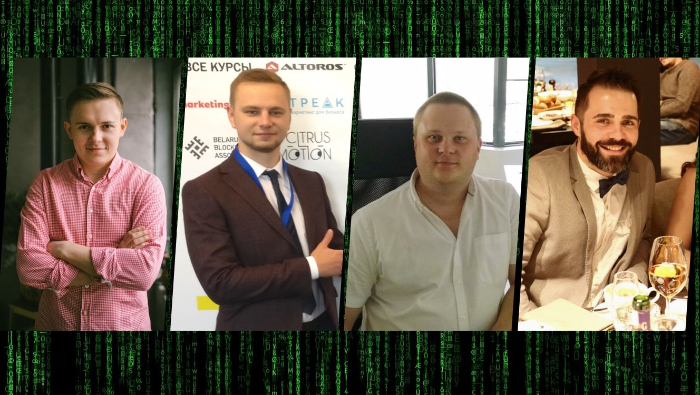
Discussion: Do Humans Expect Too Much of AI?
LEARN MORE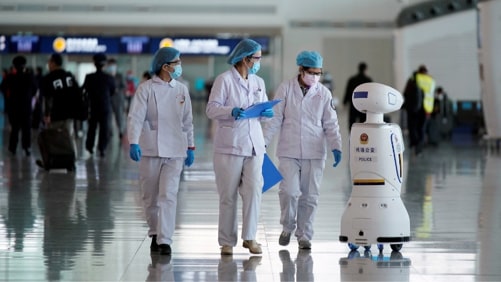